A Quantitative Analysis
This article reports the results of a series of data analyses of how recent Supreme Court nominee Brett Kavanaugh compares to other potential Supreme Court nominees and current Supreme Court Justices in his judging style. The analyses reveal a number of ways in which Judge Kavanaugh differs systematically from his colleagues. First, Kavanaugh dissents and is dissented against along partisan lines. More than other Judges and Justices, Kavanaugh dissents at a higher rate during the lead-up to elections, suggesting that he feels personally invested in national politics. Far more often than his colleagues, he justifies his decisions with conservative doctrines, including politicized precedents that tend to be favored by Republican-appointed judges, the original Articles of the Constitution, and the language of economics and free markets. These findings demonstrate the usefulness of quantitative analysis in the evaluation of judicial nominees.
Introduction
When institutions are tested and politics are divided, Americans look to the courts as the final safeguard against instability and the erosion of rights. This is especially true of the Supreme Court and sets the stakes for any new addition to the bench. With a conservative majority in the balance, the nomination to replace Justice Kennedy gives President Donald J. Trump the opportunity to define American law for the next generation.
As social scientists who use data to understand the U.S. legal system, we have analyzed the records of the short list of potential nominees. Though we can’t read their minds, new data technologies allow us to sift through a judge’s record to uncover new insights into his or her worldview and political values.
First, though judges are nominally expected to sit above the partisan fray, we find evidence that Judge Kavanaugh is highly divisive in his decisions and rhetoric. He tends to dissent and be dissented against, typically along partisan lines.
Indeed, Judge Kavanaugh is in the top percentiles of dissents, especially against Democrat-appointed colleagues. This divisiveness ramps up during election season: Kavanaugh in particular is observed disagreeing with his colleagues more often in the lead-up to elections, suggesting that he feels personally invested in national politics.
Next, we examined the writing style of Judge Kavanaugh and compared it to the writing style of recent Supreme Court Justices. We found that Judge Kavanaugh’s writing style is closest to that of Justice Samuel Alito, one of the Court’s more conservative Justices.
We then looked at some of the political and ideological features of Kavanaugh’s opinions that may be driving his motivation to dissent, and his similarity to Justice Alito. Far more often than his colleagues, Judge Kavanaugh justifies his decisions with conservative doctrines, including politicized precedents that tend to be favored by Republican-appointed judges, the original Articles of the Constitution, and the language of economics and free markets.
Finally, an exploratory sentiment analysis of his opinions shows that Judge Kavanaugh tends to speak negatively of liberalism. He also expresses dislike toward government institutions and toward working-class groups.
These results will be useful to senators and the public as they consider the nomination of Judge Kavanaugh for the Supreme Court. More generally, this essay shows that a quantitative approach can be useful in evaluating judge decision-making. This approach could be used in the future, not only for the Supreme Court, but for other judgeships.
The rest of this Essay is organized as follows. Section I describes the previous literature. Section II describes the data and empirical approach. The next sections report the results on divisiveness (Section III), text similarity to Supreme Court Justices (Section IV), political and ideological content (Section V), and sentiment associations (Section VI). These are followed by a Conclusion.
I. Previous Literature
Presidential nominations of Supreme Court Justices have generated significant scholarly interest. Some recent literature focuses on attempting to predict the ideological leanings of President Trump’s pick to replace Justice Kennedy;1 another set of work investigates the policy preferences and writing style of Trump’s most recent appointee, Justice Neil Gorsuch.2
Judicial ideology itself is the subject of a large body of literature, much of which has focused on Supreme Court Justices.3 C. Hermann Pritchett’s work on the Roosevelt Court is an early example of a quantitative approach to estimating the ideologies of individual Justices using voting data.4 A more recent, influential example is the Martin-Quinn score, which places ideology in the context of a broader judicial decision model that allows for judicial policy preferences along multiple dimensions, variance in the salience of these dimensions across individual cases, and temporal shifts in policy preferences.5 Other established approaches to estimating judicial ideology that are not reliant on vote data (and therefore are applicable prior to confirmation) include analyzing the text of editorials describing the nominees6 and using the political ideologies of the Presidents who nominate them, or the senators from the candidates’ home states, as proxies.7
Much of the above literature grappling with judicial ideology utilizes techniques that are not readily applicable to both Supreme Court Justices and judges on lower courts; political scientists and legal scholars seeking to apply uniform analysis to a broader set of courts have developed other approaches better calibrated to indicia of ideology and policy preference that are available throughout federal and state judiciaries. In The Judicial Common Space, the authors unify the approach of leveraging the ideologies of appointing presidents and candidates’ home state senators8 with Martin-Quinn scores for Supreme Court justices to provide a common analytical policy space for all Court of Appeals judges and Supreme Court Justices appointed since 1953.9 Others take a different, agency-motivated approach, using clerk ideology to estimate the ideology of judges throughout the federal judiciary.10 These authors create these “Clerk-Based Ideology” (CBI) scores by matching samples of individuals who clerked between 1995 and 2004 with population-level data on political donations and validating judges’ CBIs through a comparison to Giles et al.’s NOMINATE scores.11
Recent work by Charles M. Cameron, Jonathan P. Kastellec, and Lauren A. Mattioli uses a “characteristics approach” to presidential selection of Supreme Court nominees that formalizes presidential demand functions for a variety of nominee attributes.12 Rather than envisioning a presidential selection process singularly focused on candidate ideology and its effect on the ideological composition of the Court, the characteristics approach theorizes that presidents nominate candidates based on demand for a bundle of their desirable attributes, including ideology, experience, background, race, and gender.13 Demand for each attribute is a function of the attribute’s political returns to the president and the costs associated with placing an individual with that attribute on the Court. In addition to explicating a theory of selection that helps to explain why presidents do not always optimize their potential ideological impact on the Supreme Court,14 Cameron, Kastellec, and Mattioli apply their characteristics approach to 54 nominees and 299 shortlisters chosen by 15 presidents between 1930 and 2018.15 They find that the ratio of benefits to costs of candidate attributes is predictive of the level of ideology and policy reliability of nominees;16 moreover, both presidential interest in selection and the availability of candidates with desirable attributes has increased over time, leading in part to increasingly diverse pools of candidates.17
One thread in judicial ideology literature that is of particular relevance to an analysis of Judge Kavanaugh is the information provided by dissent behavior. Although academic observers have long found dissent behavior salient,18 a substantial body of recent literature places dissents and dissent aversion in the context of broader judicial behavior and leverages dissent-related insights to estimate judicial ideology.19 One feature of this body of literature is the attempt to explain why judges undertake dissents despite potential collegiality and effort costs incurred in dissenting. Because dissents generally result in more work for the majority, who as a rule revise their opinions in response to dissents,20 and can generate majority resentment at being critiqued, dissenting may impose collegiality costs on judges and Justices, making them less well-liked and potentially disadvantaged in attempting to get their colleagues to join them in future decisions.21 This effect is likely to be amplified by larger caseloads, which suggests that collegiality costs may be higher for Courts of Appeals judges than for Supreme Court Justices.22 The fact that judges dissent despite these costs suggests that the value that they place on the potential influence of a dissent—with its attendant reputational enhancement—and the promotion of their own views, outweighs collegiality and effort costs.23 It is not clear that the small influence enjoyed by dissents on average (as measured primarily by the very low rates of dissent citation in both the Supreme Court and Courts of Appeals),24 however, provides much of an incentive to dissent apart from “self-expressive utility.”25
How judges express themselves, in terms of vocabulary and other markers of writing style, has also generated a significant body of literature. While some contributions to this scholarship have been qualitative,26 much recent work has been quantitative.27 Nina Varsava, for instance, uses computational linguistics methods to find that Neil Gorsuch’s writing differs from that of his former Tenth Circuit colleagues, including in its level of informality, suspenseful structure, and use of qualifiers.28 Other quantitative work explores responsiveness of word choice to panel effects and reversal aversion.29
The instant piece adds to recent research using tools from machine learning and artificial intelligence to analyze judicial decision-making. Much of traditional scholarship on how legal decisions are made has been based on theoretical work, anecdotal evidence, and the analysis of academics,30 but the field increasingly benefits from robust case-level datasets.31 The availability of this data has made it possible to use machine learning tools to evaluate judicial decision making and assess whether it can be improved by assistance from algorithms.
One insight of this recent analytical literature is the extent to which legal outcomes can vary even when judges are presented with similar facts. In one example, a study documents a wide range in the probability of applicants being granted asylum from judge to judge when certain facts are held constant.32 Another example shows that changes in judges’ working conditions can impact effort and decision quality (as measured by citations), which both vary widely from judge to judge.33 Other recent studies show direct evidence of judges at least occasionally responding to extraneous factors like hunger, weather, and sporting event outcomes.34
In prediction applications, machine learning algorithms are most useful when prediction criteria are well defined and when large amounts of data are available to “train” the algorithms. Although their use in the legal system is currently limited, algorithms have been successfully employed to predict failure to appear in bail settings,35 re-arrest of violent offenders,36 and local violence for police targeting.37 Ex-post algorithmic assessment of court decisions is rarer, but at least one parole board uses such a process.38 Notably, one study has been able to predict U.S. Supreme Court decisions using only information available before the decision—at 70% accuracy.39
A handful of legal scholars have recently employed a particular machine-learning approach—topic modeling—to analyze large amounts of text from court cases. In particular, there is work that quantitatively assesses the stylistic output of the Supreme Court. One 2016 piece analyzes the entire historical body of the Court’s opinions, finding that contemporaneous Justices write more similarly to each other than to Justices of different time periods, but that stylistic heterogeneity has grown with the increasing involvement of clerks in opinion writing.40 Another piece takes a similar approach to showing that the Court’s style is growing more distinct from those of lower courts over time.41
II. Data and Empirical Approach
The data for this analysis are constructed from the full corpus of U.S. Circuit Court cases, obtained from Bloomberg Law. The analysis includes all published Circuit Court decisions for the years 1975 (the earliest year of appointment for the included judges) through 2013. For each decision, we have authoring judge and his/her co-panelists, the filing date, some metadata such as area of law, and the full text of each opinion (majority, concurring, and dissenting).
For the results, we focus on the set of judges who served on a federal Circuit Court and were later nominated for or promoted to the U.S. Supreme Court. The results are reported as figures, with the judges abbreviated as BKav (Brett Kavanaugh), NGor (Neil Gorsuch), MGar (Merrick Garland), Bork (Robert Bork), Alito (Samuel Alito), AKen (Anthony Kennedy), Soto (Sonia Sotomayor), JRob (John Roberts), RBG (Ruth Bader Ginsburg), SBre (Stephen Breyer), CTho (Clarence Thomas), and Scalia (Antonin Scalia). Except in Section V (comparison of writing style), the data consists of their Circuit Court, not Supreme Court, decisions.
The statistical regression estimates are generated using standard fixed-effects regression methods. We control for court and time specific factors, either in terms of levels or in variability. We cluster the standard errors by judge. Regression tables for all figures are available on request from the authors.
Most of the results are reported as coefficient plots, constructed as statistical differences from other judges. The marks give the average difference of the judge from the comparison group judges, while the error spikes are 95% confidence intervals summarizing the precision of the estimate. The horizontal dashed line is zero, so estimates where the confidence interval is far away from the dashed line indicate a statistically significant difference. A marker above the gray dashed line indicates a positive effect, while a marker below the gray dashed line indicates a negative effect.
While the regression methods are standard, what is new in the methodology is the adoption of recent text-based metrics of judge writing style. These include language similarity to other judges, extraction of partisan language or citations, use of economic analysis in opinions, and measuring expressed sentiment toward types of topics or social groups. Description of these methods, and citations to the relevant sources, are included along with the associated results.
The metrics that we have analyzed here are likely to be predictive of how Kavanaugh would make decisions on the Court. In unpublished work, we have used our historical data to show that these circuit-court metrics are predictive of subsequent decisions on the Supreme Court. To do this, we used the published decisions of all 26 appellate judges who sat on at least fifty circuit cases and later served on the Supreme Court from 1946 to 2016. As the outcome, we used the average conservativeness of a Supreme Court Justice’s decisions, as coded by the Supreme Court Database.42 We then looked at the bivariate correlations separately for each metric.
We found that a judge who moves from using the most Democratic language to the most Republican language is associated with a 23 percent increase in conservative votes. Moving from the most Democratic precedent citations to the most Republican precedent citations is associated with a 32 percentage point increase in conservativeness. A judge who moves from the lowest to highest rank in similarity to Judge Posner is 18 percentage points more likely to vote conservative; for economics language usage, that difference is 6 percentage points. Moving from the lowest to highest rank in vote polarization means a 25 percentage point increase in conservative vote rate, and 8 percentage points for electoral dissent rate. These differences are statistically significant.
III. Evidence of Divisiveness
We start by looking at disagreement. In Circuit Courts, cases are usually decided by panels of three judges, and sometimes there are dissents. We would like to know how Judge Kavanaugh compares, in terms of dissents, to his Circuit Court colleagues and to Supreme Court Justices.
A. Writing and Provoking Dissents
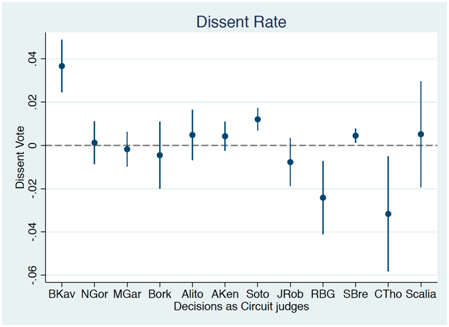
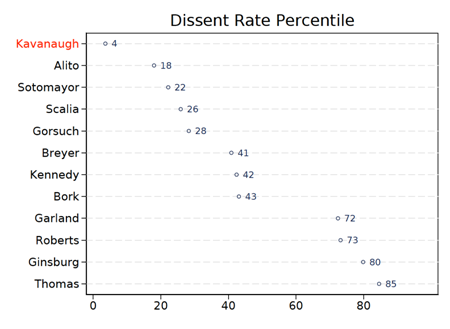
In Figure 1A, we ask whether Kavanaugh dissents more often than his colleagues. In this figure, as in most of those below, we show a coefficient plot to indicate statistical differences between the named judge and other judges on the same Circuit.43 We see that Judge Kavanaugh dissents much more often than his colleagues.
This story is strengthened in Figure 1B, which reports the percentiles for each of the judges on dissent rates. This is the rank of the indicated judge compared to the whole population of circuit court judges in this time period. Kavanaugh is ranked in the top 4th percentile for overall dissent rate.
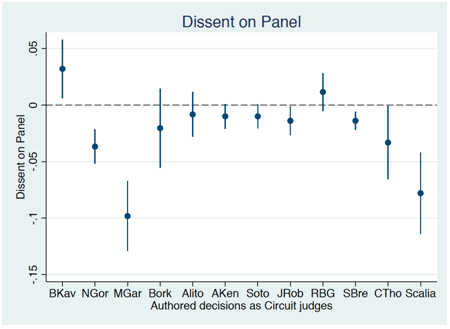
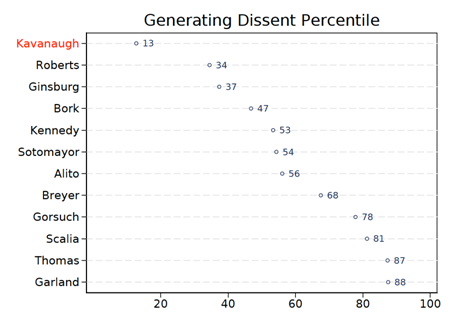
In Figures 2A and 2B, we ask a related question: When a judge authors an opinion, is it likely to provoke a dissent? We see again that in the case of Judge Kavanaugh, the answer is yes. When Kavanaugh authors an opinion, it is highly likely that a dissent will be filed against him, compared to his colleagues. As seen in Figure 2B, Kavanaugh is in the top 13th percentile for generating dissents. In contrast, Gorsuch and (especially) Garland generated fewer dissents than average.
B. Partisan Dissents
Next we look at how these dissent tendencies vary with the partisan affiliation of judges. While federal judges do not have an official party label, we can make strong inferences about their partisan ties based on the party of the President who initially appointed them. So in our case, Reagan, Bush (I or II), and Trump nominees are considered Republicans: Kavanaugh, Gorsuch, Bork, Alito, Kennedy, Roberts, Thomas, and Scalia. Clinton/Obama nominees are considered Democrats: Garland, Sotomayor, Ruth Bader Ginsburg, and Breyer. We ask whether a judge is more likely to dissent when paired with political opponents.
For this analysis, the regression is the same as in the previous section, except that the treatment variable is interacted with a dummy variable equaling one for when the other two members on the panel are from the opposing political party.44 So a positive coefficient means the judge dissents more often when co-panelists are from the political opposition. The idea is that judges who tend to dissent only against judges appointed by the opposing party’s president are engaging in “Partisan Dissents” or “Vote Polarization.”45
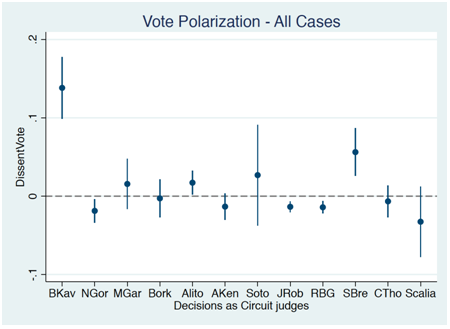

Figures 3A, 3B, and 3C report this analysis. First, in Figure 3A, we see that Kavanaugh is relatively polarized in his dissents. This means he tends to dissent more often when sitting with two Democratic-appointed co-panelists. Figure 3B shows that Kavanaugh, like Alito, tends to dissent against Democrats in Due Process cases. Figure 3C indicates that Kavanaugh is a true outlier on this metric, ranking in the top 1st percentile of judges based on partisan dissents.
C. Election-Season Dissents
Another way that dissents can provide evidence of political motives is whether they respond to external political factors. A previous paper shows that circuit court judges tend to dissent more in the run-up to presidential elections, consistent with a (perhaps subconscious) political cheerleading motive.46 In this section, we ask how Kavanaugh and other Supreme Court nominees respond on this margin: Do they tend to dissent more in the months leading up to a presidential election?
This analysis again builds on the basic dissent analysis from above, with the addition that the treatment variable is interacted with a dummy variable equaling one for February through October of a presidential election year.47 A positive coefficient means that a judge dissents more than usual (that is, compared to his/her normal baseline) during election seasons.
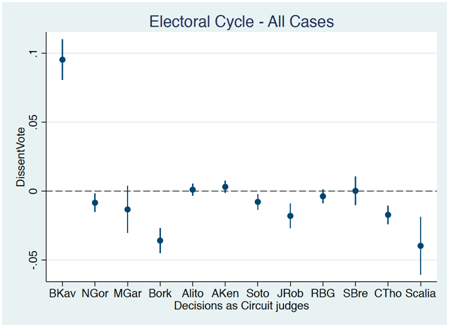
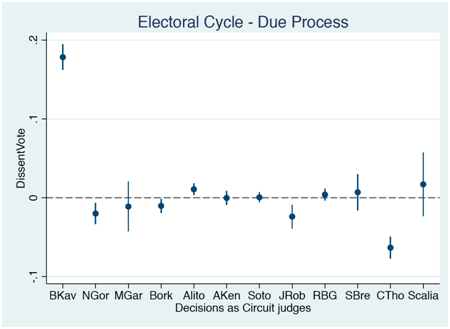
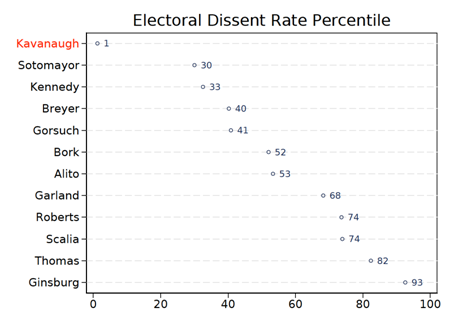
We see in Figure 4A that, indeed, Kavanaugh does get fired-up during election seasons. In the months leading up to presidential elections, Kavanaugh is more likely to dissent relative to his baseline level of dissents (which, we have highlighted above, is already higher than that of his colleagues). Figure 4B shows that the electoral dissent effect happens especially for Due Process cases. And again, as illustrated in Figure 4C, Kavanaugh is a true outlier on this metric, ranking in the top 1st percentile. Sonia Sotomayor, the second-highest Supreme Court nominee on this metric, is in the 30th percentile.
Electoral dissent is potentially a problematic behavioral tendency. It is indicative of susceptibility to extraneous factors, raising the question of what other behavioral factors—beyond political—might affect a judge’s decisions. Do behavioral factors become relevant in settings where judges are closer to indifference?
IV. Comparison of Writing Style to Supreme Court Justices
Next we will start looking into the linguistic content of Judge Kavanaugh’s opinions. As he is a nominee for the Supreme Court, we would like to ask what type of Justice he might be. To do this, we construct a geometric representation of his writing style, as expressed in the language of his authored opinions. We then look at the distance (or closeness) of this writing style to the geometric representations of the case portfolios for each recent Supreme Court Justice.
We use a text analysis technique, which we described in a recent paper,48 that allows us to measure the similarity of language across judges. The technique works as follows. Document vectors for each case are constructed using the Doc2Vec implementation in Python Gensim.49 The vectors are de-meaned by court, topic, and year, to try to isolate ideological content, and then averaged by judge. Judges are compared by the cosine similarity (geometric closeness) of the vectors.
Figure 5 shows the similarity of Kavanaugh’s writing to a set of recent Supreme Court Justices. The figure provides coefficient plots from judge-level regressions, where the outcome is similarity to the named Supreme Court Justice, and the coefficient is on a dummy variable for Kavanaugh. A mark further to the right means a higher similarity of case language to the indicated Supreme Court Justice. A mark to the left means lower language similarity.

As can be seen in Figure 5, Kavanaugh is most similar to Justice Samuel Alito, a Bush appointee with a reputation as a conservative. Interestingly, while Kavanaugh was a former Kennedy clerk, he is not relatively similar to Kennedy in how he writes his opinions.50 These rankings give some insight into what kind of Justice Kavanaugh would be if confirmed.
V. Political and Ideological Features of Opinions
In this section we dig deeper into the political and ideological content of Judge Kavanaugh’s opinions. These results will provide some evidence on the set of decision motives that are driving Judge Kavanaugh’s tendency to dissent, and his similarity to Alito.
A. Language and Precedent Partisanship
What are the doctrinal sources of ideological polarization in the judiciary? One idea is that the language used, or the authorities cited, might be informative about political views. To investigate this issue, we use the partisan association of text and citations to measure the degree of polarization among these judges.
First, we would like to measure the use of partisan language in each case. To do so, we use a dictionary of partisan phrases, as described in the working paper by Ash, Chen, and Lu,51 which provides a ranking of those phrases associated more with Democrat-appointed judges and those phrases associated more with Republican-appointed judges. A case with more of these phrases could then be considered to be relatively politicized, and similarly with a judge who uses more of these phrases. The method is based on a recent paper that analyzes polarization in congressional speeches.52
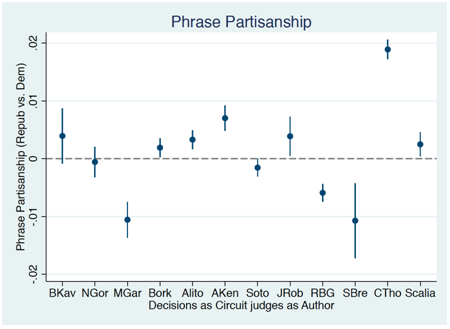
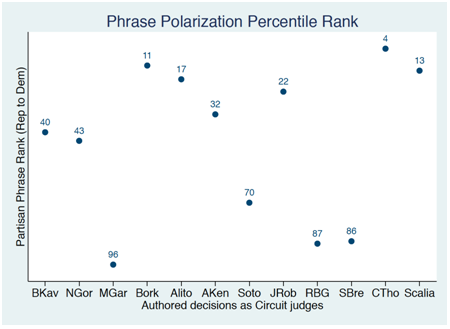
Figures 6A and 6B show how the Supreme Court nominees stack up in terms of the partisanship in their language.53 In these coefficient plots, a positive coefficient means the judge prefers language distinctive of a Republican, while a negative coefficient indicating preference for Democrat-distinctive language. We can see in Figure 6A that Kavanaugh is more polarized than average, but it is not quite statistically significant. In the percentile rankings (Figure 6B), he is beat by Chief Justice Roberts and Justice Thomas.
Another way that a case could exhibit partisanship is in its selection of previous cases that are cited as references. Anecdotally, it is well recognized that some precedents are preferred by Republicans and others are preferred by Democrats. The working paper by Ash, Chen, and Lu54 provides a data-driven ranking of precedents along this margin, where, based on the citations in an opinion, one could potentially predict the political party of the authoring judge. In our regressions using precedent partisanship as an outcome, a positive coefficient means that the judge uses Republican-distinctive precedents, while a negative coefficient indicates the use of Democrat-distinctive precedents.
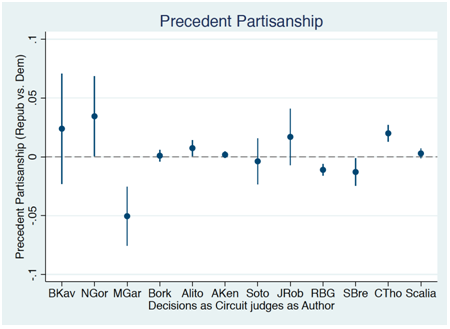

In Figure 7A, we see a positive coefficient for Judge Kavanaugh, but it is noisy; Justice Gorsch is more polarized in his selection of precedents. Judge Garland, on the other hand, uses citations and phrases more often associated with Democrat appointees. But in Figure 7B, we can see that Kavanaugh is in fact quite polarized in terms of his ranking among all judges. He is in the 15th percentile on precedent polarization.
In the case of language, it could be that Kavanaugh is polarized in a dimension not easily detected along traditional markers of party lines or economics. As Stanford Law Professor Bernadette Meyler has written, “in cases involving politically controversial issues, [Kavanaugh] tends to acknowledge the views of both sides while stating something to the effect that these political considerations can’t weigh into his decision and he is instead bound by Supreme Court precedent.”55
B. Citations to the Constitution
A major factor underlying ideological citation could be Originalism—the legal philosophy that advocates attention to founding-era documents and their original meanings. To investigate this possibility, we looked at citations to the Articles of the U.S. Constitution, in particular Articles II and III. These articles are interesting because they refer to the powers of the presidency (Article II) and the judiciary (Article III). Conservative jurists tend to cite Article II as favoring expanded executive power, including a narrow reading of civil liberties in criminal law, terrorism, and related contexts. Judges tending to cite Article III may favor more power given to the judiciary. In both cases, a focus on Article II and Article III (rather than Article I, which concerns Congress) may be interpreted as favoring the less democratic branches of government.
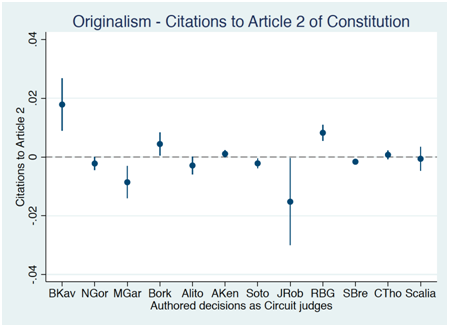
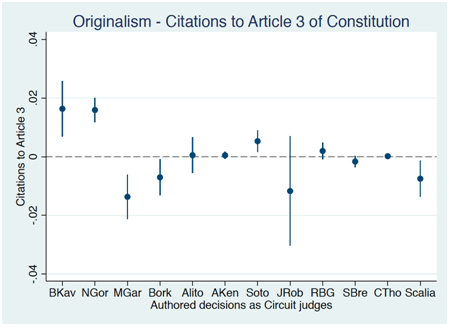
For this part of the analysis, outcomes are whether a case cites Article II or Article III of the Constitution.56 These results are reported in Figures 8A and 8B. Judge Kavanaugh cites both Article II and Article III more than the other jurists analyzed. In comparison, Judge Garland was less inclined to cite either of the two Articles. According to this measure, Kavanaugh has tended to use Originalist reasoning in his opinions.
C. Use of Economic Analysis
Another major source of conservative ideology in the judiciary is law and economics. In ongoing work, we have shown that economics training and economics ideology is an important source of conservative, anti-regulatory decision-making in the courts.57 Here, we look at how President Trump’s nominee compares on the use of economics language in his opinions.
We provide two measures of the use of economics language in opinions. These measures are constructed using the document vectors for cases described in Section V, supra. As described in our working paper,58 the cases are represented in a joint vector space with words, where, for example, cases related to “economics” would be closer in the vector space to the vector for the word “economics.” Here, therefore, we use the closeness to the economics vector as a flexible measure of the use of economics language and concepts in opinions. The second measure is the similarity of case language to Judge Richard Posner, a former circuit court judge and leader in economic analysis of law. This Posner metric is constructed the same way that the similarity scores to Supreme Court Justices were constructed in Section V, supra.
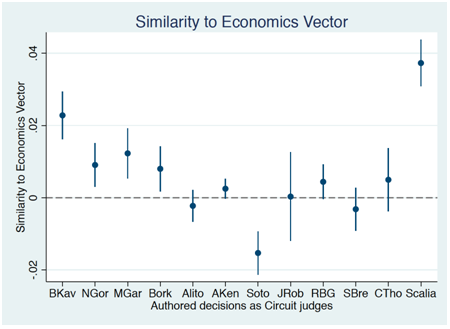
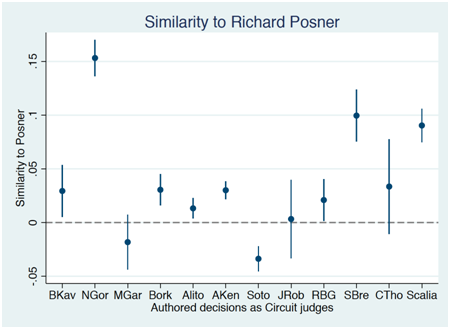
Figures 9A and 9B provide regression results for the economics measures.59 Judge Kavanaugh has been writing opinions that reflect economics language (Figure 9A). In addition, Kavanaugh is similar to Posner relative to his colleagues (Figure 9B). Gorsuch, Bork, and Scalia also tend to rank highly on these metrics.
VI. Sentiment Toward Groups and Institutions
To better understand the differences among these judges, we explore the use of sentiment analysis to analyze their written opinions. The goal is to measure the use of positive or negative sentiment when the judges discuss various topics.
As detailed in a working paper,60 we approach this problem using document vectors (also using Doc2Vec) for each sentence in each case. We then compute the vector similarity of each sentence to a set of targets and a set of sentiment words. The targets include liberal, congress, federal, labor, and farmer. The sentiment words include positive language (warm, favorable, good) and negative language (cold, unfavorable, bad). For a case, the sentiment toward a target is the covariance between sentence similarity and target similarity by sentence.
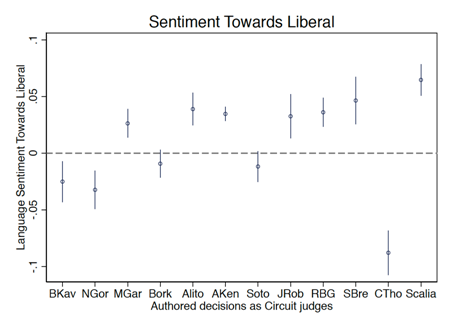
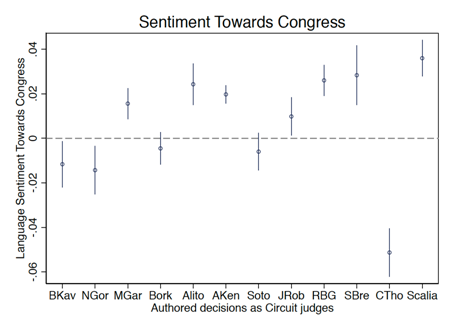
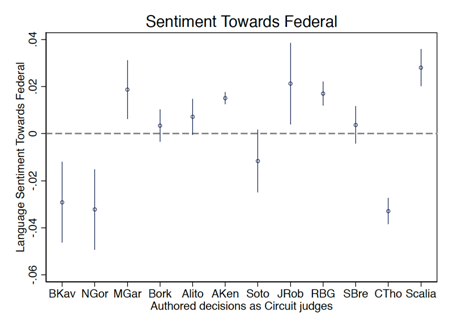


The figures are coefficient plots from case-level regressions, where the outcome is sentiment toward the named target.61 In Figure 10A, we see that Kavanaugh expresses negative sentiment towards liberals. This provides some additional evidence from language content about the ideological preferences indicated in the previous results.
In Figures 10B and 10C, we see that Judge Kavanaugh expresses negative sentiment towards Congress and the Federal Government. These “anti-government” attitudes are consonant with ideas about the dangers of regulation and a large welfare state.
Finally, Figures 10D and 10E show that Judge Kavanaugh expresses negative sentiment towards labor unions and farmers. This could reveal some negative views for the working class.
An important caveat with these sentiment metrics is that they are something of a black box, and can be driven by many implicit associations in language. They also seem to be quite noisy, as, for example, Justices Scalia and Thomas tend to be on wildly different sides of the sentiment coin for some of the targets. The more experimental language metrics, such as these sentiment associations, should be interpreted and used with caution.
Conclusion
In sum, Kavanaugh is not your average judge. Compared to his circuit court colleagues, and to other recent Supreme Court Justices, Kavanaugh is an outlier on a range of margins. The Trump Administration, the U.S. Senate, and the American people should reckon with these facts and figures in the coming weeks.
This analysis is useful not just for the confirmation decision on Judge Kavanaugh, but also for thinking ahead to future judicial nominations. This approach could be used for future Supreme Court nominations of Circuit Court judges, and similar comparisons could be made for other judgeships. We hope that adding more quantitative evidence into judicial nominations could result in a more meritocratic judicial selection process and a better-functioning judiciary.